r2 calculation using devsq and prediction multiple is an essential topic in statistics. It helps us understand how well a model predicts outcomes. If you want to know if your model is doing a good job, look at R².
Simply put, R² tells you how much of the changes in your data can be explained by your model. When we use DEVSQ, we can determine how much the data points are spread out. Combining this with prediction multiple helps us see if our model is adequate.
What is R² Calculation?
R² calculation, also known as the coefficient of determination, is a way to measure how well a model fits the data. It helps us see if our predictions are good or not. The value of R² ranges from 0 to 1, where a score close to 1 means our model explains the data very well, while a score close to 0 means our model does not explain the data effectively. This concept is essential in statistics, especially for tasks like r2 calculation using devsq and prediction multiple
Understanding R² is helpful in many fields, such as science, business, and economics. It allows people to make better decisions based on data. For example, if a company wants to know how well a new product will sell, it can use R² to evaluate the accuracy of its predictions. Through r2 calculation using devsq and prediction multiple, the company can make smarter choices.
Additionally, knowing how to calculate R² is beneficial for students and professionals alike, as it enhances their skills in data analysis. By applying R², anyone can determine if their model works well with the data they have. In this way, R² becomes a valuable tool for verifying predictions and understanding patterns in data.
Understanding DEVSQ in Statistics
DEVSQ, short for “deviation squared,” is a statistic that shows how much individual data points differ from the average, and it’s vital for r2 calculation using devsq and prediction multiple methods. When calculating DEVSQ, we square the differences between each data point and the mean, emphasizing the larger differences. A higher DEVSQ value indicates that the data points are widely spread out, showing greater variability within the data set.
Using DEVSQ is especially important when conducting r2 calculation using devsq and prediction multiple. This calculation gives insight into how well a model can predict outcomes by showing the variance within the data. By including DEVSQ, we gain a better understanding of the predictive accuracy of our model.
Why Use Prediction Multiple?
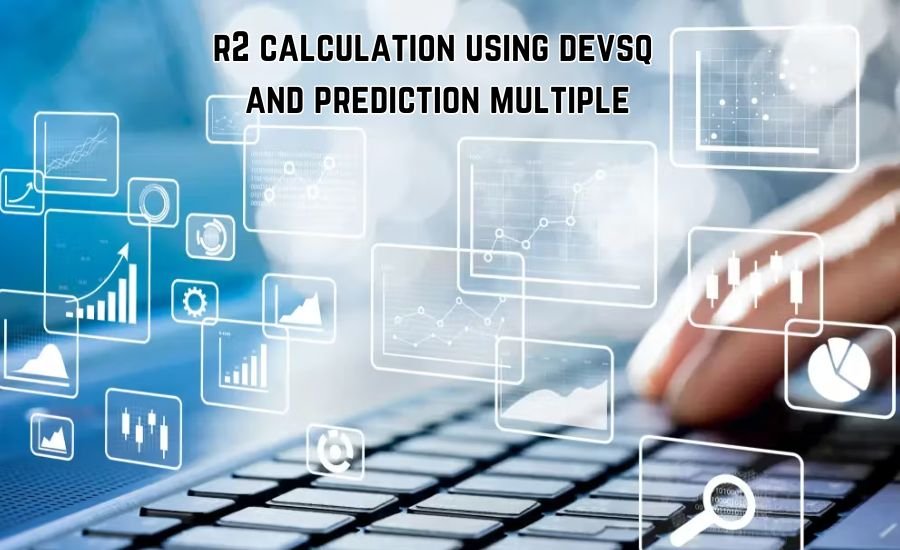
Prediction multiple is a powerful concept in data analysis, where we use multiple factors to predict an outcome. Instead of relying on a single variable, we analyze several different factors together. For example, if we want to predict a student’s performance, we might examine their study time, attendance, and homework scores. This approach, known as prediction multiple, helps us build a more accurate prediction model.
Incorporating prediction multiple is essential for improving the R² calculation using DEVSQ. By including more variables, we capture a fuller picture of the data, which strengthens our model. The R² calculation using DEVSQ becomes more precise with the added information, as this statistical measure helps us understand the variance in our predictions. With prediction multiple, we improve our models, making them more reliable.
Prediction multiples have practical applications in fields such as marketing and healthcare. Marketers use this approach to optimize strategies for reaching different audiences. Meanwhile, doctors apply prediction multiple to assess patient health by examining multiple indicators. In each case, prediction multiple and R² calculation using DEVSQ lead to more accurate, dependable results.
How R² Calculation Works
R² calculation works by comparing the total variation in the data and examining how much of this variation is explained by the model. The formula for R² involves DEVSQ and the total sum of squares (TSS). First, we find the TSS, which tells us how much the data points vary from the mean. Then, we calculate how much our model improves this variation. In essence, R2 calculation using DEVSQ and prediction multiple lets us see how well our model fits the data.
When we calculate R², we can see if our model is valid. A high R² value shows that our model explains a large portion of the data—great news! However, if R² is low, we might need to rethink our approach. Perhaps we are missing essential factors in our predictions or need to refine our R2 calculation using DEVSQ and prediction multiple.
In practice, R² calculation is often done using computer software. Programs can quickly handle large amounts of data, making it easier for researchers and analysts to understand their results. With the right tools, calculating R² becomes simple and efficient.
The Importance of R² in Data Analysis
The importance of R² in data analysis cannot be overstated. It serves as a quick check to see how well a model performs. When people use data to make decisions, they want to be confident in their results. A high R² score gives them that confidence. It shows that the model is reliable. For instance, when performing an r2 calculation using devsq and prediction multiple, a higher score indicates a better fit between the model and the data.
R² is also essential because it helps in comparing different models. If two models predict the same outcome, you can use R² to see which is better. The model with the higher R² is usually preferred. This is helpful in many fields, including science and business. By conducting an r2 calculation using devsq and prediction multiple, analysts can identify which model provides the most accurate predictions.
In summary, R² is a critical measure in data analysis. It helps us check our predictions and compare models. By understanding R², anyone can make better decisions based on data. This is why R² is a valuable tool for students and professionals alike.
Steps for R² Calculation Using DEVSQ
To calculate R² using DEVSQ, follow these simple steps. First, collect your data. This can be any set of numbers related to your research. Next, find the average of your data points. After that, calculate DEVSQ by squaring the differences between each data point and the mean.
Once you have DEVSQ, you must find the total sum of squares (TSS). This involves adding up all the squared differences. After calculating both DEVSQ and TSS, you can discover R². The formula is R² = 1 – (DEVSQ / TSS). This will give you your final R² score.
Completing these steps lets you understand how well your model fits the data. If your R² is high, you can be more confident in your predictions. On the other hand, a low R² means you might need to adjust your model or look for additional factors. This process of r2 calculation using devsq and prediction multiple helps you evaluate the effectiveness of your model in explaining the variability in your data.
Using Prediction Multiple in Your Analysis
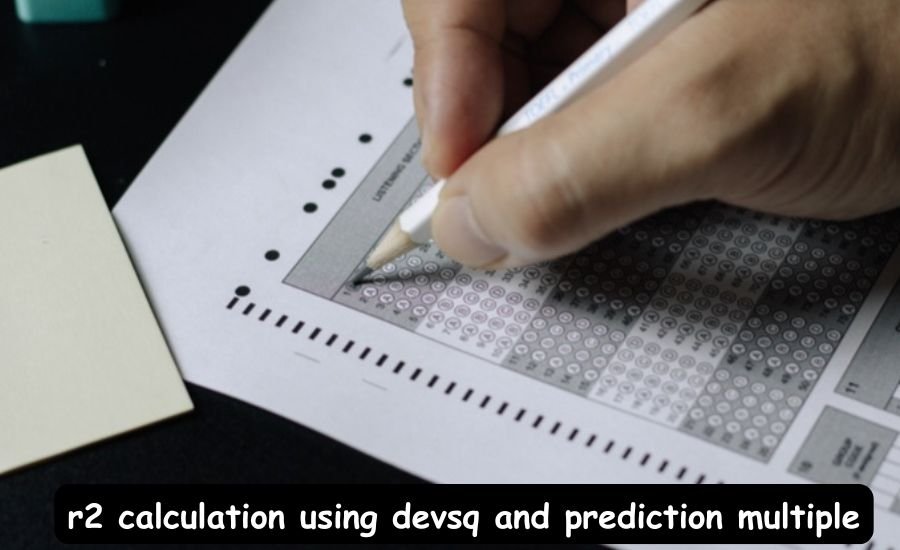
When you use prediction multiple in your analysis, you must gather data on various factors. What might influence the outcome you are trying to predict? For example, if you want to predict a student’s grades, consider factors like study habits, attendance, and even their home environment. Understanding these variables is essential, especially when performing an r2 calculation using devsq and prediction multiple measure the model’s effectiveness.
Once you have collected data on these factors, you can analyze it using statistical software. Many programs allow you to input multiple variables and see how they affect the outcome, helping you create a more comprehensive and reliable model. The r2 calculation using devsq and prediction multiple
enables you to evaluate how well your model fits the data, showing you the proportion of variance explained by your predictors.
Including prediction multiple leads to better insights. When you look at many factors together, you can see patterns you might miss when looking at just one. This makes your predictions stronger. It’s like having a complete puzzle instead of just a few pieces. By leveraging the r2 calculation using devsq and prediction multiple, you can enhance the accuracy of your predictions and gain a deeper understanding of the factors at play.
Examples of R² Calculation Using DEVSQ and Prediction Multiple
Let’s look at some examples to make this more transparent. Suppose we want to predict how well students perform in school based on different factors. We could collect data on study time, attendance, and homework completion. After gathering this information, we calculate R² using DEVSQ to see how well our model explains the data. The r2 calculation using devsq and prediction multiple will help us determine the effectiveness of the factors we’ve chosen.
If we find a high R² value, we can say that our model is good at predicting student performance. It means that the factors we considered help explain the students’ grades. On the other hand, if R² is low, we might need to think about different aspects. Maybe we didn’t include essential elements like teacher support or parental involvement, which could be critical to understanding student success. The r2 calculation using devsq and prediction multiple allows us to refine our model by considering these additional variables.
In another example, businesses can use R² to predict sales. By considering factors such as marketing spending, seasonality, and customer reviews, they can calculate R². This helps companies understand what drives sales and improve their strategies. The key is to use the correct data and include multiple variables in the analysis. By performing the r2 calculation using devsq and prediction multiple, businesses can gain insights into how various factors influence sales performance and make informed decisions based on this analysis.
Common Mistakes in R² Calculation
When calculating R², people sometimes make common mistakes. One mistake is forgetting to check the assumptions of the model. Ensuring that your data meets the requirements for R² to be meaningful is essential. If the data is not appropriate, your results might be misleading.
Another mistake is using too few variables in the analysis. R² works best when you include multiple factors. You might miss important information if you only look at one or two. This can lead to a lower R² score than expected.
Lastly, some people need to consider the context of their data. R² values can vary by field or type of study. A high R² in one area may not be good enough in another. Always keep the context in mind when interpreting your results. This will help you avoid misinterpretations and improve your analysis.
How to Improve Your R² Score
Improving your R² score is possible with some strategies. First, consider adding more variables to your model. When you include more relevant factors, your predictions may become more accurate. This often leads to a higher R² score.
Another way to improve R² is to look for outliers in your data. Outliers are data points that are very different from the rest. They can affect your R² score negatively. By analyzing and possibly removing these outliers, your model may fit better.
Lastly, ensure you are using a suitable model for your data. Sometimes, there may be better choices than a linear model. Trying different types of models can lead to better results. By experimenting and refining your approach, you can increase your R² score and make more robust predictions.
Comparing Models Using R²
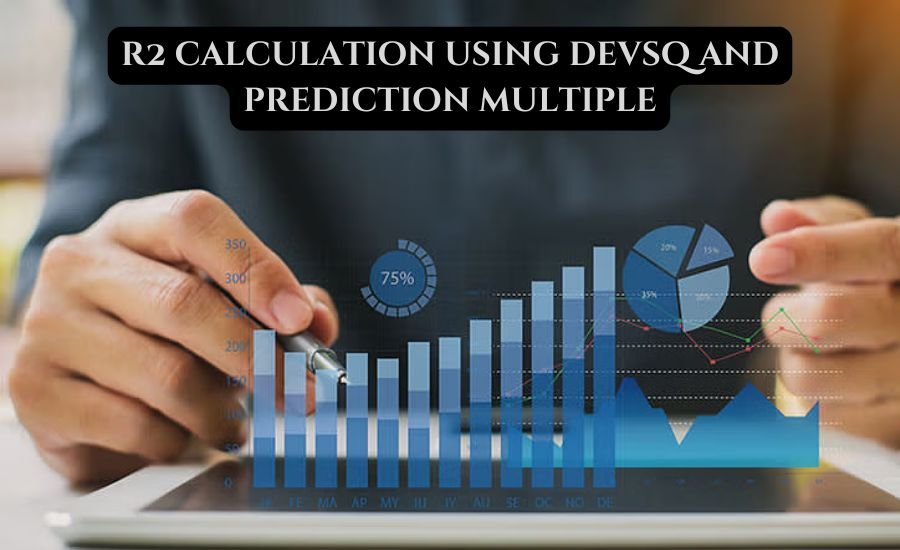
Comparing models using R² is a valuable practice in data analysis. When you have multiple models predicting the same outcome, R² helps you determine which model is better. The model with the higher R² score usually explains the data more effectively.
For example, calculating R² for both can guide your choice if you have a simple linear and a more complex model. It may be the better option if the complex model has a much higher R². This comparison allows you to select the most reliable model for your analysis.
However, it’s important to remember that R² is not the only measure to consider. Sometimes, a slightly lower R² model could be simpler and easier to use. Balancing simplicity and accuracy is critical when choosing your model for predictions.
R² Calculation: A Simple Guide
R2 calculation using devsq and prediction multiple might sound complex, but it can be simple with the proper steps. Start by collecting your data and finding the average. Next, calculate DEVSQ by squaring the differences between each data point and the mean. Once you have this, you can see the total sum of squares (TSS).
After you have both DEVSQ and TSS, use the formula: R² = 1 – (DEVSQ / TSS). This will give you your R² score. A higher score means your model fits the data well, while a lower score indicates room for improvement.
By following these steps, anyone can calculate R². Whether you are a student, a researcher, or just curious about data, understanding R² is beneficial. It is a handy tool for checking how well your predictions work. r2 calculation using devsq and prediction multiple helps you gauge the effectiveness of your predictive models.
Real-Life Applications of R² Calculation
R² calculation has many real-life applications. Teachers can use it in education to see how different factors affect student performance. They can understand what influences success by analyzing data from test scores, homework completion, and attendance. By employing r2 calculation using devsq and prediction multiple, educators can gain insights into which factors significantly impact student outcomes, helping improve teaching methods.
In business, companies use R² to predict sales and customer behavior. They can understand what drives sales by examining factors like marketing campaigns, pricing strategies, and customer feedback. Utilizing r2 calculation using devsq and prediction multiple allows businesses to refine their strategies, leading to better decision-making and improved profits.
Moreover, healthcare professionals apply R² to study patient outcomes. By analyzing factors such as treatment types, age, and lifestyle, they can see what works best for patients. This helps create effective treatment plans and improve patient care. Overall, R² calculation is a valuable tool across many fields, enhancing understanding and effectiveness in various applications.
Conclusion: Why R² Matters in Statistics
In conclusion, r2 calculation using devsq and prediction multiple is essential in statistics. It helps us measure how well our models predict outcomes. With R², we can assess the quality of our predictions and improve our analyses. This makes it a crucial tool for students, researchers, and professionals.
Understanding concepts like DEVSQ and prediction multiple enhances our ability to analyze data. By using these tools together, particularly in r2 calculation using devsq and prediction multiple, we can create more robust models. This leads to better insights and more reliable predictions.
In today’s data-driven world, knowing how to calculate and interpret R² is vital. It empowers individuals to make informed decisions based on solid data analysis. As you explore the world of statistics, remember the importance of R² and its applications in everyday life.
Must Read: Understanding-stla-unlevered-free-cash-flow
FAQs
Q: What is R² calculation?
A: R² calculation measures how well a statistical model explains data variability. It ranges from 0 to 1, with a value close to 1 indicating a good fit.
Q: How do you calculate R²?
A: To calculate R², find the total sum of squares (TSS) and the sum of squared deviations (DEVSQ). Use the formula R² = 1 – (DEVSQ / TSS).
Q: What does a high R² value mean?
A: A high R² value (close to 1) means that the model explains a large portion of the variability in the data, indicating a good fit.
Q: What is DEVSQ?
A: DEVSQ stands for deviation squared. It is the sum of the squared differences between each data point and the mean, showing how data varies.
Q: Why is prediction multiple important?
A: Prediction multiple considers multiple factors to improve prediction accuracy. It provides a more comprehensive view of what influences the outcome.
Q: How can R² be used in business?
A: In business, R² helps analyze sales data and marketing effectiveness, allowing companies to make informed decisions based on reliable predictions.
Q: What are common mistakes when calculating R²?
A: Common mistakes include neglecting model assumptions, using too few variables, and misinterpreting the context of R² values.
Q: How can I improve my R² score?
A: To improve your R² score, add more relevant variables, check for and address outliers, and ensure you are using the appropriate model for your data.